Threat Modeling AI/ML: Building Secure and Responsible Systems
The rapid advancements in Artificial Intelligence (AI) and Machine Learning (ML) have brought about a revolution across various industries, offering immense potential and convenience. However, with this power comes the critical need for robust security measures to address the unique risks associated with these technologies.
Traditional security practices often fall short when dealing with AI/ML systems, demanding specific approaches to safeguard against potential threats. This blog post explores some of the key challenges in securing AI/ML systems and proposes strategic solutions to mitigate them:
Understanding the Unique Challenges:
Vulnerability to Malicious Data: ML models can struggle to distinguish between genuine and malicious data, making them susceptible to manipulation through poisoned or adversarial data sets.
Overreliance and Lack of Transparency: Blindly trusting AI/ML outputs without understanding the underlying decision-making process can lead to biased or incorrect results.
Limited Forensic Capabilities: The “black box” nature of certain AI systems hinders the ability to understand their decision-making process and audit their actions, especially in high-stakes scenarios.
Building Secure and Responsible AI/ML Systems:
Designing for Resilience and Discretion: Integrating security principles into the design phase of AI systems is crucial. This includes incorporating mechanisms to detect and mitigate potential attacks while respecting privacy and ethical considerations.
Bias Management and Recognition: Identifying and addressing biases within AI systems is essential to ensure fair and responsible AI development and deployment.
Enhancing Malicious Data Detection: Implementing robust anomaly detection techniques and data validation procedures can significantly improve the ability of AI systems to discern and filter out malicious data.
Developing Forensic Capabilities: Building transparency and accountability into AI systems requires the development of robust forensic tools that can explain and audit decision-making processes.
Securing Sensitive Information: Implementing stringent data security measures and access controls is vital to protect sensitive information handled by AI systems.
Moving Forward: Strategic Solutions:
Establishment of Specialized Security Bodies: Creating dedicated AI/ML-focused penetration testing and security review bodies can provide crucial expertise to assess and mitigate potential threats.
AI Security Training for Developers: Equipping developers with specialized training on AI security best practices can foster a culture of security within the development process.
Hardening ML Algorithms: Research into techniques to harden ML algorithms against manipulation and poisoning of training data is essential to ensure their robustness.
Centralized Libraries and Continuous Learning: Establishing centralized libraries for ML auditing and forensics tools while continuously analyzing evolving attack patterns are crucial for maintaining strong defenses.
By acknowledging the unique challenges and implementing strategic solutions like those outlined above, we can work towards building secure and responsible AI/ML systems, fostering trust and maximizing the positive potential of these transformative technologies.
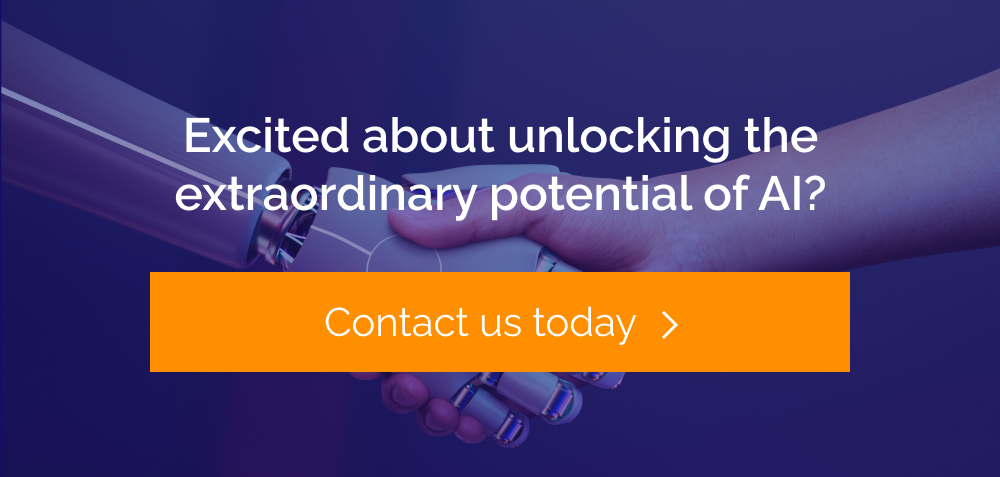